As the world seeks to transition towards cleaner and more sustainable energy sources, wind power has emerged as a formidable contender in the race to combat climate change and reduce our dependence on fossil fuels. Wind plants, with their majestic turbines dotting the landscapes, have become iconic symbols of this shift toward renewable energy.
But harnessing the power of the wind is no simple feat. The efficient operation of a wind plant demands intricate monitoring, predictive analysis, and a profound understanding of the complex forces of nature. This is where machine learning steps onto the stage, ready to transform the world of wind plant analytics.
In this article, we explore the dynamic and evolving role of machine learning in wind plant analytics. We will explore how this innovative technology is not only optimizing energy production but also revolutionizing the way we harness and manage wind energy.
Harnessing nature’s power with precision
The ability to accurately harness the power of nature is a critical component in wind energy sustainability. Wind plants are designed to harness the kinetic energy of flowing air and transform it into electrical power. These plants need to be closely monitored and adapted to varying wind patterns, weather patterns, and operational challenges in order to maximize efficiency.
Traditionally, wind plant analytics have relied on historical data and simple statistical models to predict energy production and address potential issues. This approach provides the foundation for wind plant management, but it has limitations in the face of the complex, ever-changing dynamics of wind and weather.
This is where machine learning steps in, offering the capacity to analyze vast datasets in real-time and make decisions that were once thought to be the exclusive domain of human operators. By training algorithms on historical data and continuously updating them with real-time information, machine learning models can uncover hidden patterns, anticipate maintenance needs, and optimize the performance of wind turbines in ways that were previously inconceivable.
Predictive insights for performance optimization of wind plants
One of the primary contributions of machine learning to wind plant analytics is the ability to provide predictive insights. These go beyond historical trends and consider current conditions, allowing for more accurate and immediate decision-making.
Machine learning algorithms can predict not only when maintenance is needed but also which specific components require attention. These algorithms analyze sensor data, weather forecasts, and historical performance to find anomalies that may indicate potential issues such as rotor blade imbalances or wear and tear of turbines. This level of accuracy guarantees the effective allocation of maintenance resources, reducing costs and downtime.
Furthermore, taking into account variables like wind speed, direction, and turbulence, machine-learning algorithms are able to forecast energy production with greater accuracy. This empowers wind plant operators to optimize grid integration and energy trading, increasing revenue and reducing waste.
Real-world applications of machine learning in wind plant analytics
The implementation of machine learning into wind plant analytics is no longer a theoretical concept; it is a reality that reshapes how we produce and manage wind energy. Let us take a closer look at some of the real-world applications of machine learning in this field:
Condition-based maintenance and anomaly detection
Machine learning algorithms are adept at analyzing data from various sensors placed on wind turbines. These algorithms monitor factors such as power output, temperature, and vibration patterns to forecast when specific components will need maintenance. The algorithms can quickly spot unusual patterns in turbine behavior or sensor data, indicating potential problems or inefficiencies. This condition-based approach reduces downtime and lowers operational costs, by ensuring that maintenance efforts focus on components showing signs of wear.
The Predictive module of GPM Horizon is a clear real-world example of machine-learning algorithms being effective. This is how it works:
- Assets connect to GPM Horizon and the tool analyzes available historical data. Deep learning-based Digital Twins are trained on normal operational data using 10-minute resolution Supervisory Control and Data Acquisition (SCADA) and conditional monitoring system (CMS) vibration data.
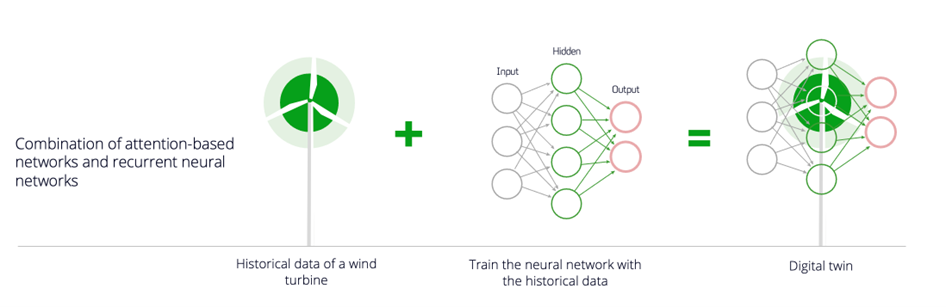
- Anomalies and their severities are identified by the error between the expected outputs from digital twins and real outputs and/or vibration pattern recognition.
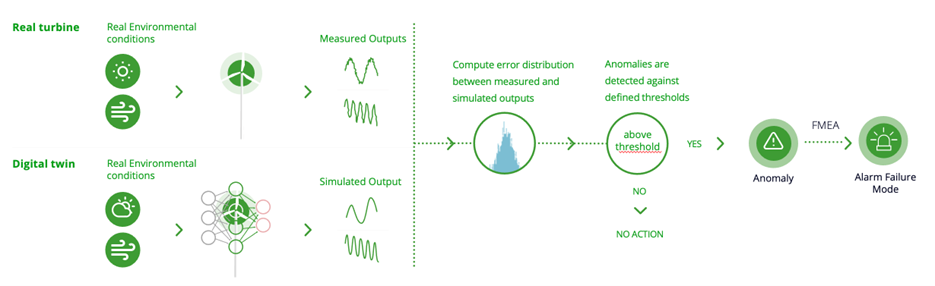
- Anomalies are fed into a Failure Mode & Effect Analysis (FMEA) matrix developed by combining the know-how of our data scientists, technology specialists and wind energy experts, to provide the most probable failure modes for each anomaly. These anomalies and the resulting possible root causes are then automatically saved to a list.
- An automatic retraining, based on the feedback field results from the site inspections, enables deep learning and FMEA probabilistic models to reduce the number of false positives, and to adjust severity levels and failure mode suggestions.
The Predictive module of GPM Horizon has successfully detected various types of anomalies: gearbox failures, obstructions in the cooling system, generator bearing overheating, lubrication system issues and many more.
The combination of the machine-learning models for automatic issue identification and the expertise of the Human-in-the-Loop (physical basis) allows our system to successfully help our clients in the maintenance of their wind assets and will continue to do so.
Improved energy production forecast
Accurate energy production forecasts are critical for effective grid integration and energy trading. Machine learning models, powered by vast datasets and real-time weather information, can make precise predictions about the energy output of a wind plant. This information empowers operators to optimize energy delivery and sales, making wind energy a more reliable and profitable resource.
GPM Horizon’s Forecast module uses machine-learning algorithms to create a park digital twin, that can predict hourly power production for the next 7 days, based on historical data and meteorological forecast from different external providers. As a result, we can achieve much lower imbalance scores, predict production better, and give more accurate information to asset managers and traders.
Example of forecast imbalance comparison for August 2023:
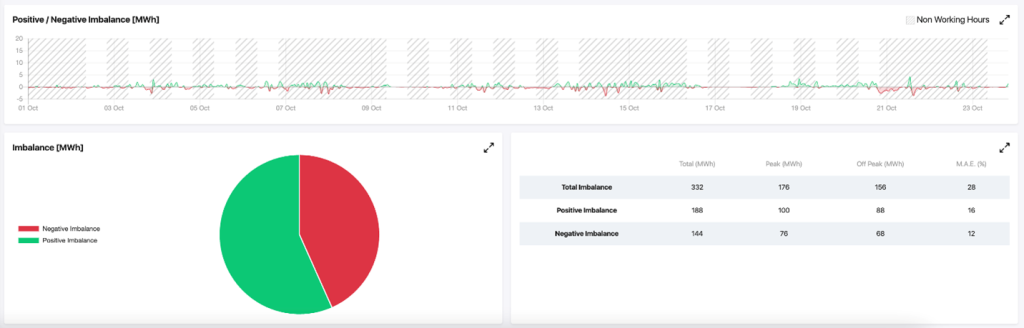
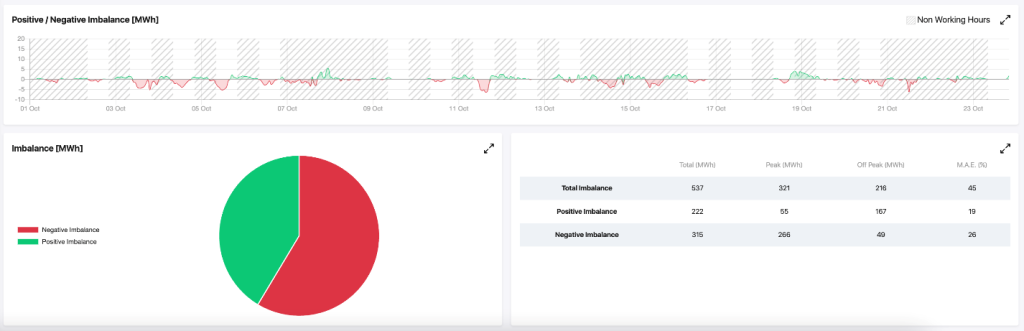
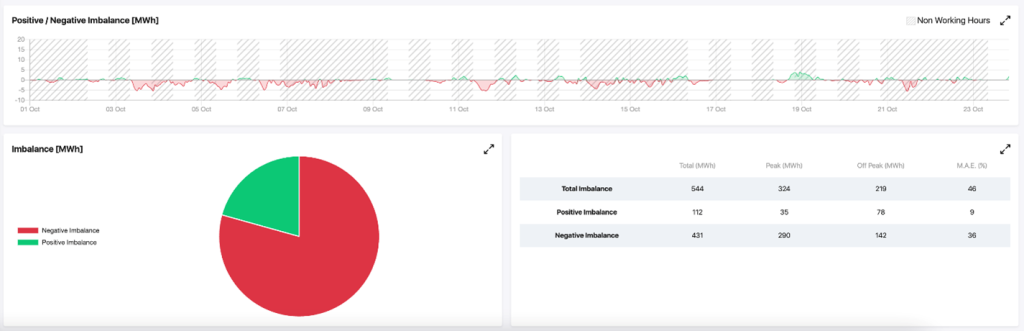
Turbine performance optimization
Machine learning can continuously monitor the performance of individual wind turbines and adjust their settings in real-time. By analyzing data from sensors on each turbine, these algorithms can make split-second decisions to optimize blade angles, rotor speeds, and other parameters to capture the maximum available energy.
Wind farm layout optimization
The optimal layout of wind turbines within a wind farm significantly impacts energy production. Machine learning can assess numerous factors, including terrain, wind patterns, and environmental conditions, to determine the most efficient layout for a given location.
Environmental impact mitigation
Machine learning also contributes to minimizing the environmental impact of wind plants. By analyzing wildlife movement patterns and environmental conditions, machine learning models can help operators implement measures to reduce harm to local ecosystems.
These applications are just a glimpse of the potential for machine learning in wind plant analytics. With continuous advancements in technology and data collection, the role of machine learning in optimizing wind plant operations is expected to grow, making wind energy a more reliable and efficient contributor to our sustainable energy future.
At GPM, we are constantly working on using machine-learning algorithms to help our clients and find real applications for it aimed at optimizing asset performance, reducing costs, and improving operational efficiency.
Opportunities and Challenges in the Integration of Machine Learning
Machine learning offers numerous opportunities for optimizing wind plant production. While the integration of machine learning into wind plant analytics holds great promise, it is not without its challenges. As with any groundbreaking technology, there are obstacles to overcome and opportunities to seize. Here, we explore both the challenges and the potential benefits:
Opportunities
- Increased efficiency: machine learning leads to more efficient wind plant operations, reducing downtime, improving energy production, and lowering operational costs.
- Environmental impact reduction: machine learning helps wind plants operate in a way that minimizes their impact on local ecosystems and wildlife.
- Enhanced reliability: predictive maintenance and real-time adjustments made possible by machine learning result in increased wind turbine and wind farm reliability.
- Energy market optimization: accurate energy production forecasts enable better grid integration and energy trading, enhancing the profitability of wind energy.
- Technological advancements: as machine learning technology evolves, it opens new possibilities for further optimization and innovation in the wind energy sector.
Challenges
- Data quality and quantity: machine learning models require substantial amounts of high-quality data for training. Wind plants can produce vast quantities of data, but ensuring their quality and relevance is a significant challenge.
- Algorithm complexity: machine learning models are complex and challenging to implement, requiring expertise in both machine learning and wind energy operations.
- Maintenance costs: deploying machine learning systems can incur additional costs, particularly in terms of data collection and model development.
- Data privacy and security: the sensitive nature of data collected from wind plants raises concerns about data privacy and security, which need to be carefully addressed.
- Regulatory compliance: the integration of machine learning may require wind plant operators to comply with new regulatory standards, calling for additional resources and effort.
A greener and more efficient tomorrow
The integration of machine learning into wind plant analytics is a testament to our commitment to a greener and more efficient energy landscape. By combining advanced data analytics with the power of renewable wind energy, we are not only shaping the future of wind plant operations but also contributing to global sustainability.
As we continue to harness the capabilities of machine learning, we open the door to further advancements in the wind energy sector. We anticipate even more efficient and reliable wind plant operations, reduced downtime, and increased energy production. These advancements not only strengthen the profitability of wind energy, but also make it a more attractive and accessible resource for communities and industries worldwide.
Machine learning bridges nature’s power with technology’s efficiency, optimizing wind plant operations for efficient wind energy management. This makes wind energy a cornerstone of renewable energy future, mitigating climate change and promoting a healthier planet. By guiding wind energy, we are on the brink of a brighter, cleaner, and more sustainable future.